Bridging Information Gaps in Industrial Data-Based Production
In today's competitive manufacturing world, data-driven decision-making is no longer an option, it's essential. Customers demand both quality and on-time delivery, leaving no room for error or improvised methods. Instead, smart manufacturers rely on insights gathered from operational systems across the front and shop floor for informed decision-making.
Yet, 20% of manufacturers admitted in a recent survey by Dassault Systèmes that they often make poor decisions due to lacking, untrusted, or inaccessible data. On the flip side, the same survey found that 70% or more respondents experienced significant business improvements when their manufacturing data was reliable and accessible.
End-to-end control and visibility of the manufacturing cycle require filling the data gaps between the front-office planning, actual production, quality assurance, and final shipment. The key to success lies in planning well and being aware of any production delays or quality issues that might ruin your plans. In this way, immediate action can be taken to rectify issues and prevent small problems from escalating.
For instance, SAY Plastics has improved its on-time delivery rates, nearing 100%, by integrating dashboards, advanced production tracking, and alert systems into their enterprise resource planning (ERP) system. This setup enables them to identify bottlenecks, quickly adjust schedules, and optimize resource allocation to meet deadlines.
Two common gaps reported in the survey were production performance and quality control. Connecting management systems in these areas with the business's primary manufacturing operating system is crucial to close these data gaps. Companies like SIGN Fracture Care have automated their quality inspection processes using their ERP system's quality modules. This has not only made inspections more efficient but also ensured regulatory compliance.
Effective data collection, integration, and utilization is the driving force behind closed-loop data-driven decision making. Implementing a unified control architecture, real-time monitoring, and automated feedback mechanisms is key to achieving this goal. By integrating production, process monitoring, and quality assurance systems, manufacturers can create truly data-driven operations that improve efficiency and overall performance.
Building a Closed Loop:
- Unified Control Systems: Connect injection molding machines, robots, and auxiliary equipment under a single control system to enable synchronized operations and real-time data sharing. Standardize communication using software-scalable industrial controllers (PLCs with OPC UA support) for interoperability.
- Real-Time Process Monitoring: Collect data from IoT-enabled sensors on variables like temperature, pressure, and cycle times, directly feeding them to analytics platforms for instant anomaly detection. Implement predictive analytics using AI/ML models to identify deviations based on live process parameters.
- Quality Assurance Integration: Integrate vision systems or spectrometers to perform real-time quality checks, linking results directly to process parameters. Incorporate closed-loop quality adjustments that modify machine settings based on quality metrics to preserve tolerances.
- Interoperability & Open Standards: Use OPC UA and MQTT to create a seamless data flow from shop floor to cloud and cloud to enterprise systems (ERP/MES). Ensure vendor-agnostic compatibility by adopting open field buses like Profinet.
- Data-Driven Feedback Loops: Program controllers to autonomously adjust processes when thresholds are breached (e.g., increasing pressure when a defect is detected). Continuously improve production schedules by aggregating historical data and refining AI models.
This closed-loop approach ensures self-optimizing production lines, where data from every stage directly informs process adjustments, quality control, and resource allocation.
- A unified control system should be implemented, connecting injection molding machines, robots, and auxiliary equipment under a single control system to enable synchronized operations and real-time data sharing.
- Real-time process monitoring is crucial, collecting data from IoT-enabled sensors on variables like temperature, pressure, and cycle times, directly feeding them to analytics platforms for instant anomaly detection.
- To ensure effective quality assurance, vision systems or spectrometers should be integrated to perform real-time quality checks, linking results directly to process parameters and implementing closed-loop quality adjustments.
- Embracing open standards like OPC UA and MQTT is essential for a seamless data flow from shop floor to cloud and cloud to enterprise systems (ERP/MES), ensuring vendor-agnostic compatibility by adopting open field buses like Profinet.
- Lastly, data-driven feedback loops should be programmed into controllers, enabling them to autonomously adjust processes when thresholds are breached and continuously improve production schedules by aggregating historical data and refining AI models.
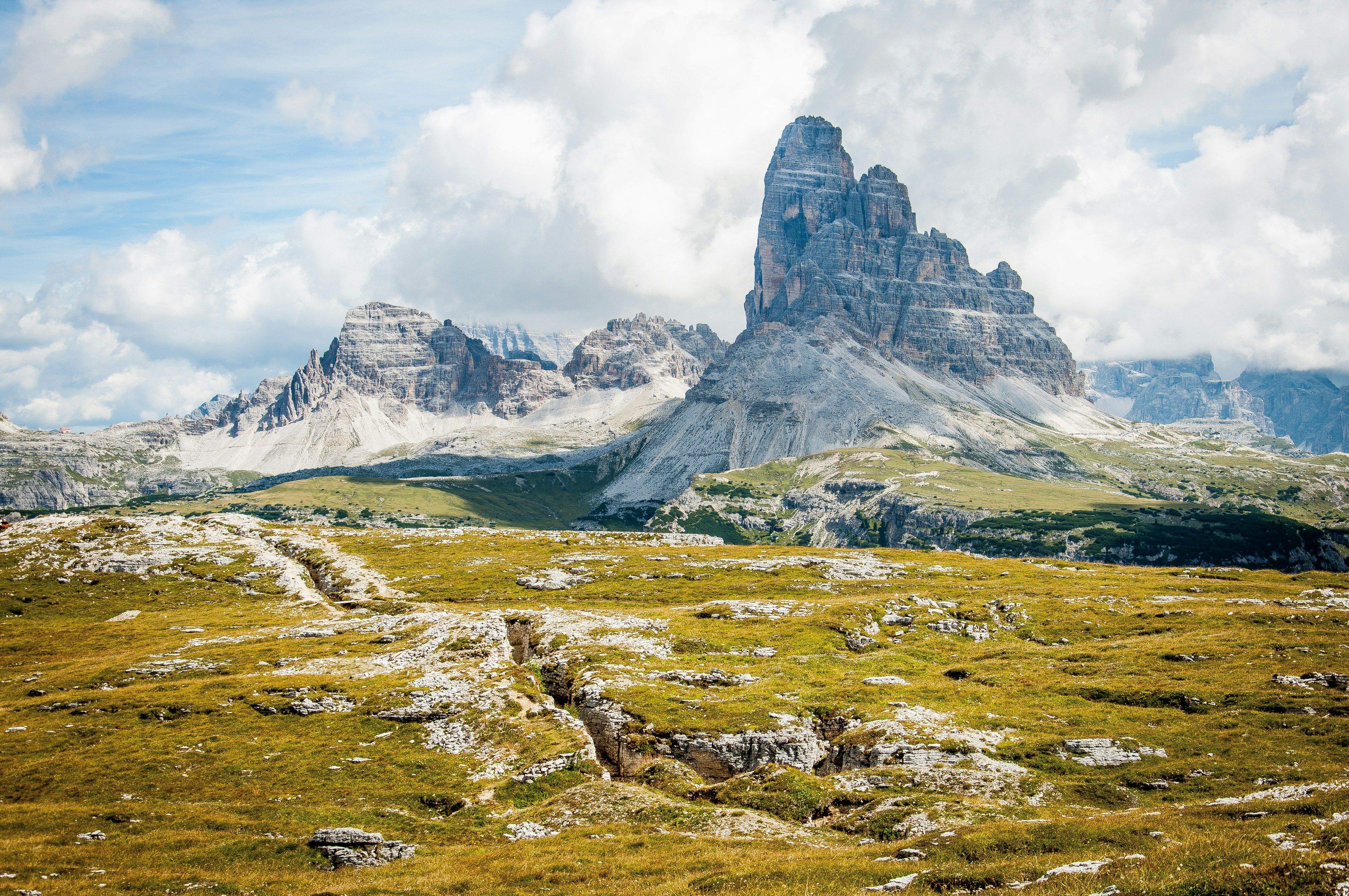
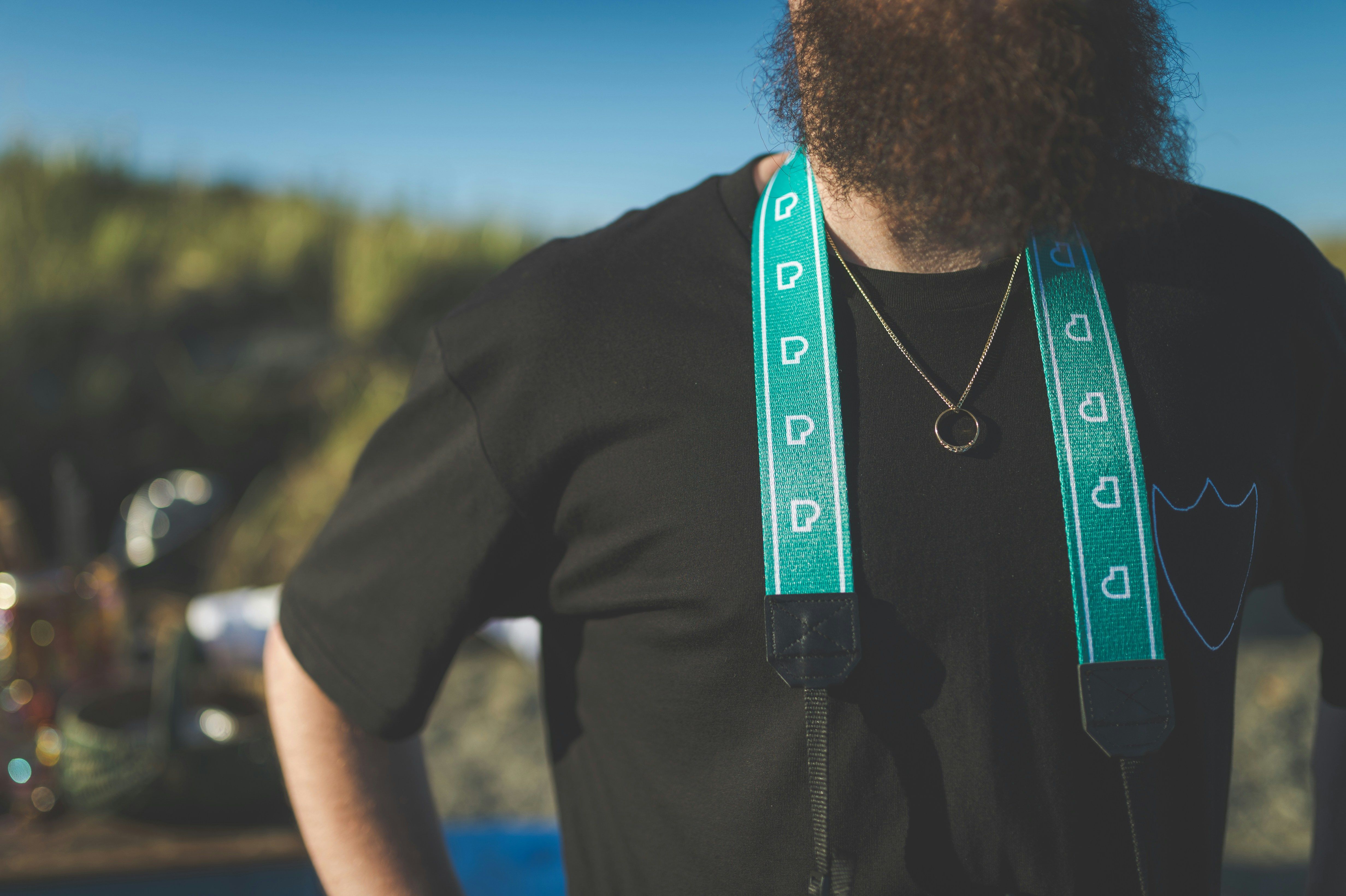
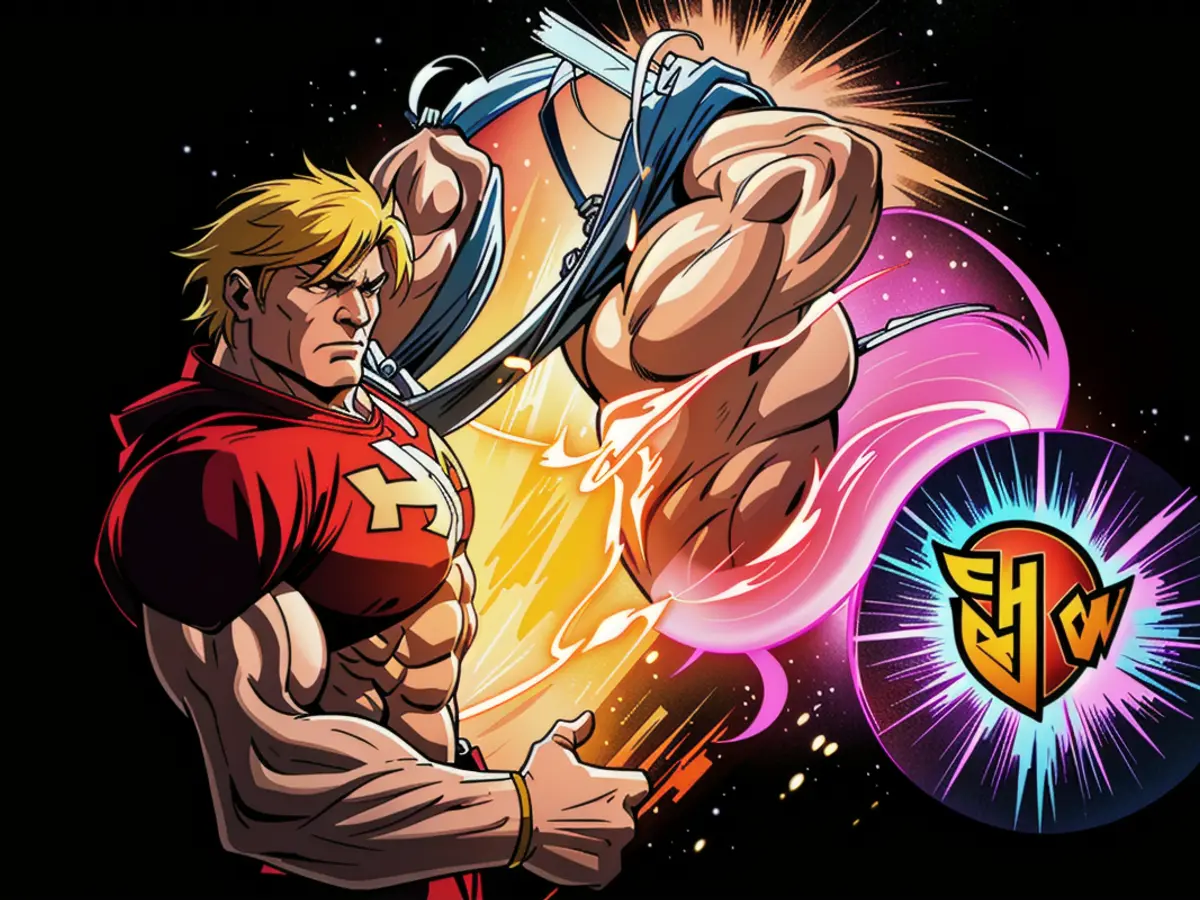